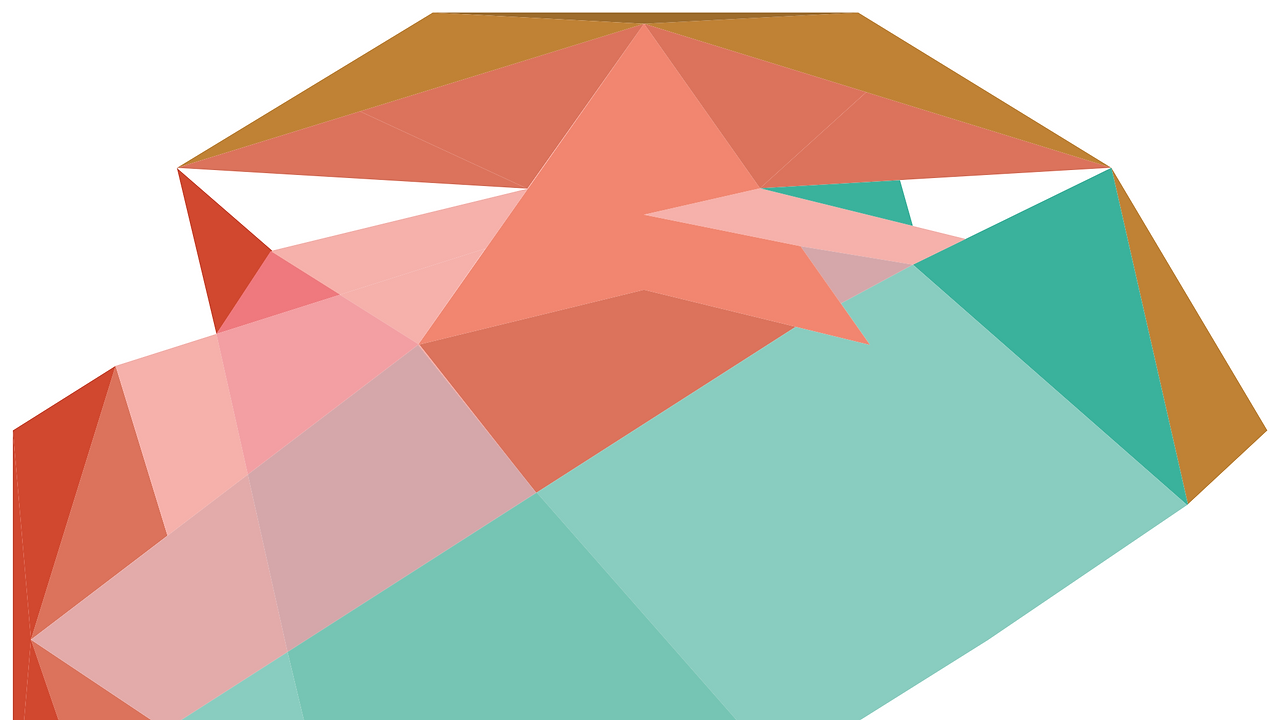
3D Flow Reconstruction Using Conic Sections And Learning-Based Image Segmentation
C. Tsalicoglou, T. Rösgen
Institute of Fluid Dynamics, ETH Zurich, Switzerland
DOI:
Extending 3D Particle Streak Velocimetry (3D-PSV) with conic section matching constraints for volumetric flow reconstruction allows the measurement of flows in large volumes using lower frame rates than typically required for 3D-PTV, with fewer reconstruction ambiguities. Streak segmentation is tackled using a state-of-the-art instance segmentation Convolutional Neural Network (CNN), highlighting the potential of learning-based methods to solve vision-based tasks in experimental fluid dynamics. The curved streaks are processed with an optimization-based conic section reconstruction approach, which results in fewer reconstruction ambiguities compared to linear streaks or individual endpoint models and enables the direct and accurate reconstruction of the curved pathlines.
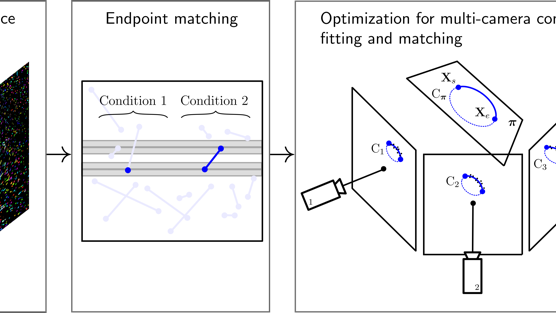